CELEHS Research
Translational Data Science Methodology
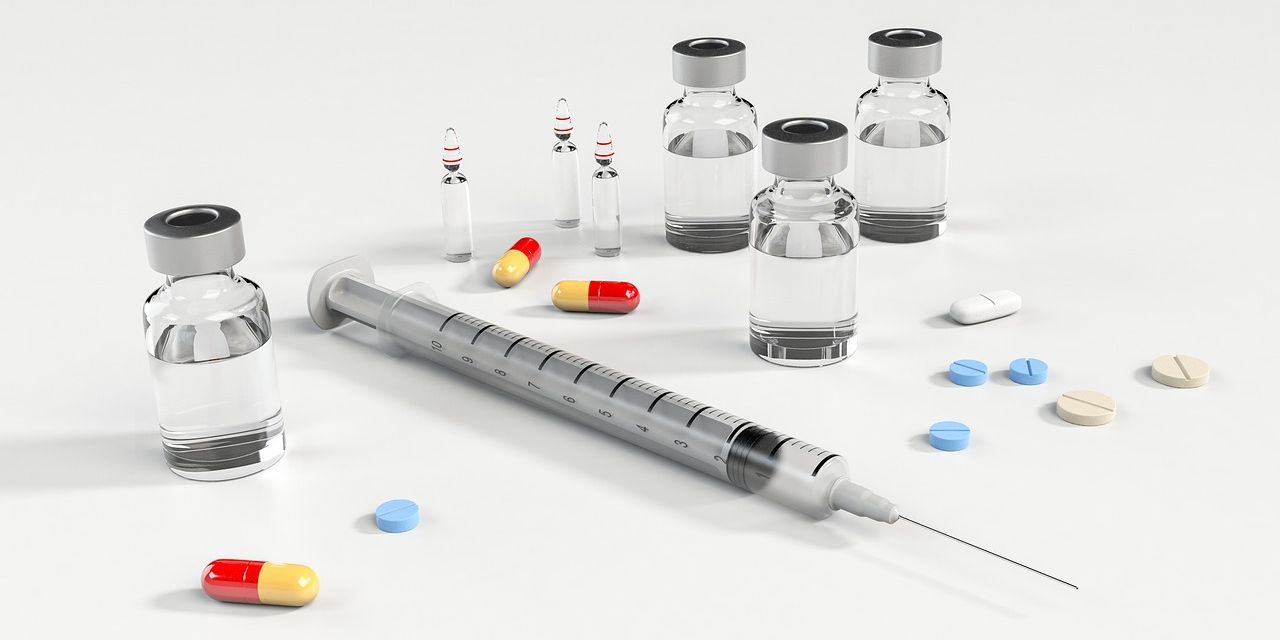
21st Century Clinical Trials
Strategies to make RCTs more feasible, efficient, pragmatic and reflective of real world settings.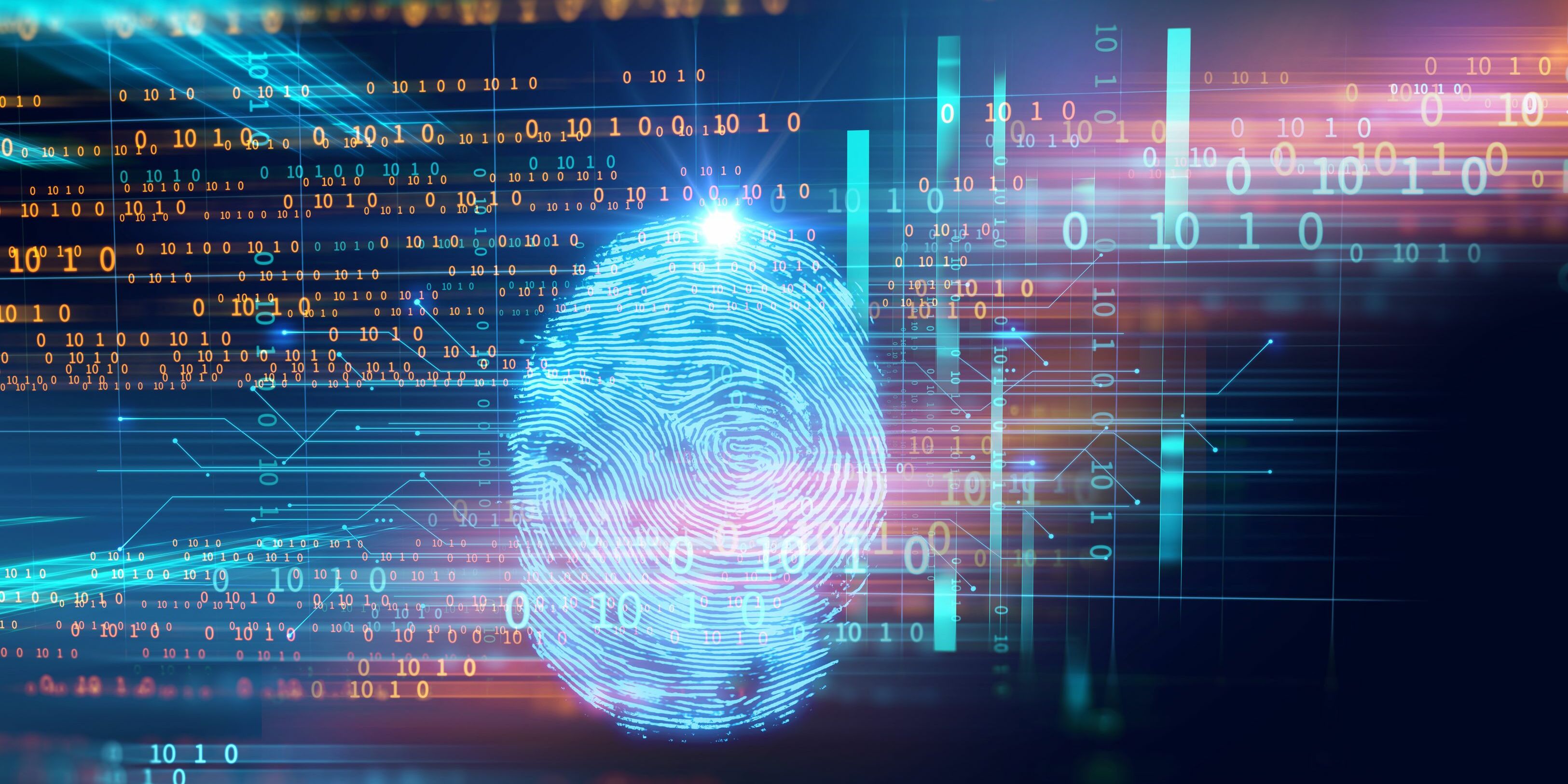
High Dimensional Inference
Strategies to detect signals, perform efficient estimation and derive prediction rules using high dimensional data.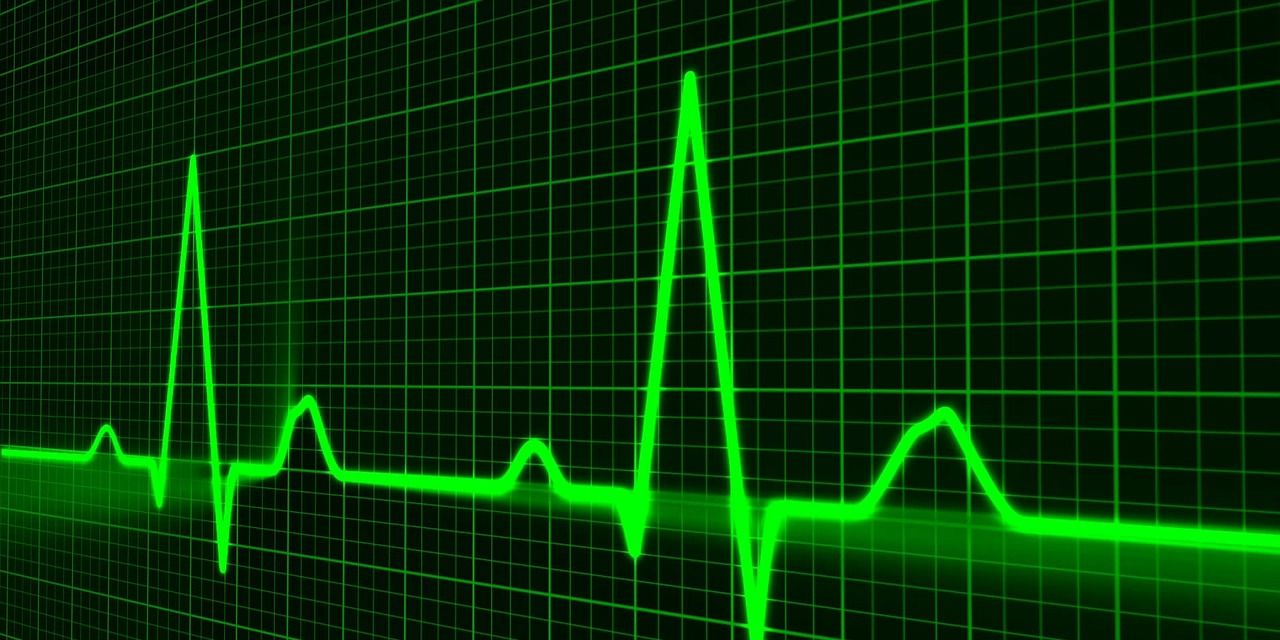
Predictive Analytics
Methodologies to derive prediction rules for disease risk and treatment response at individualized level.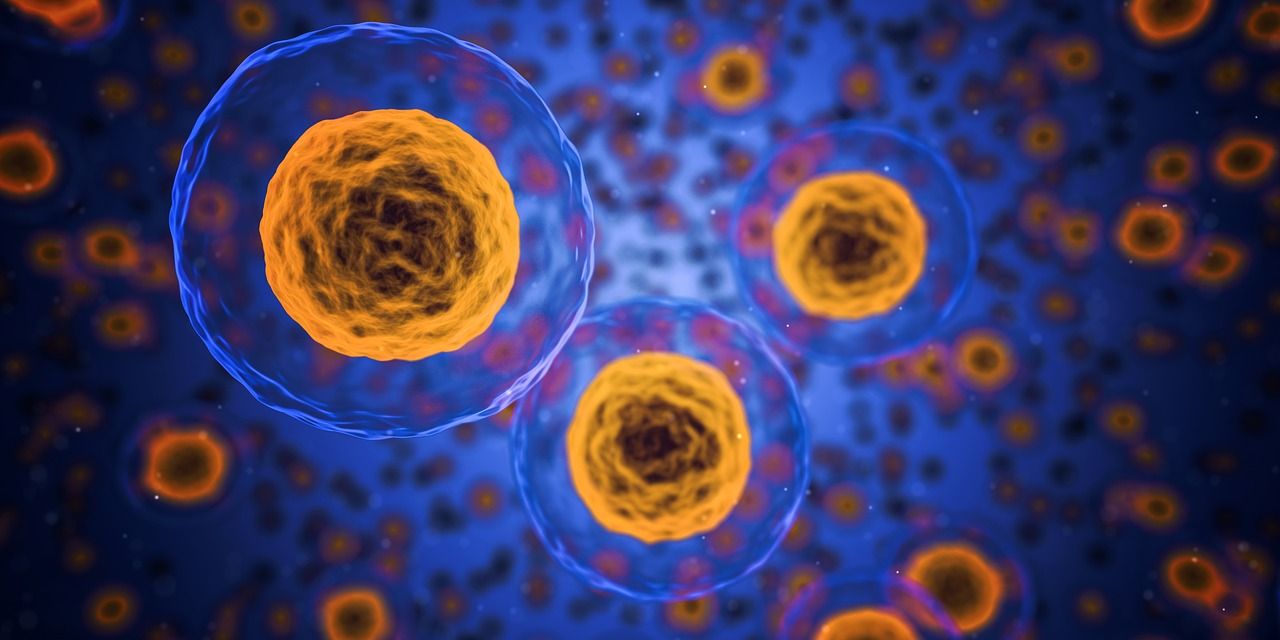
Real World Evidence
Leveraging large scale noisy electronic health records and claims data to infer about causal treatment effects.Portable Knowledge Systems
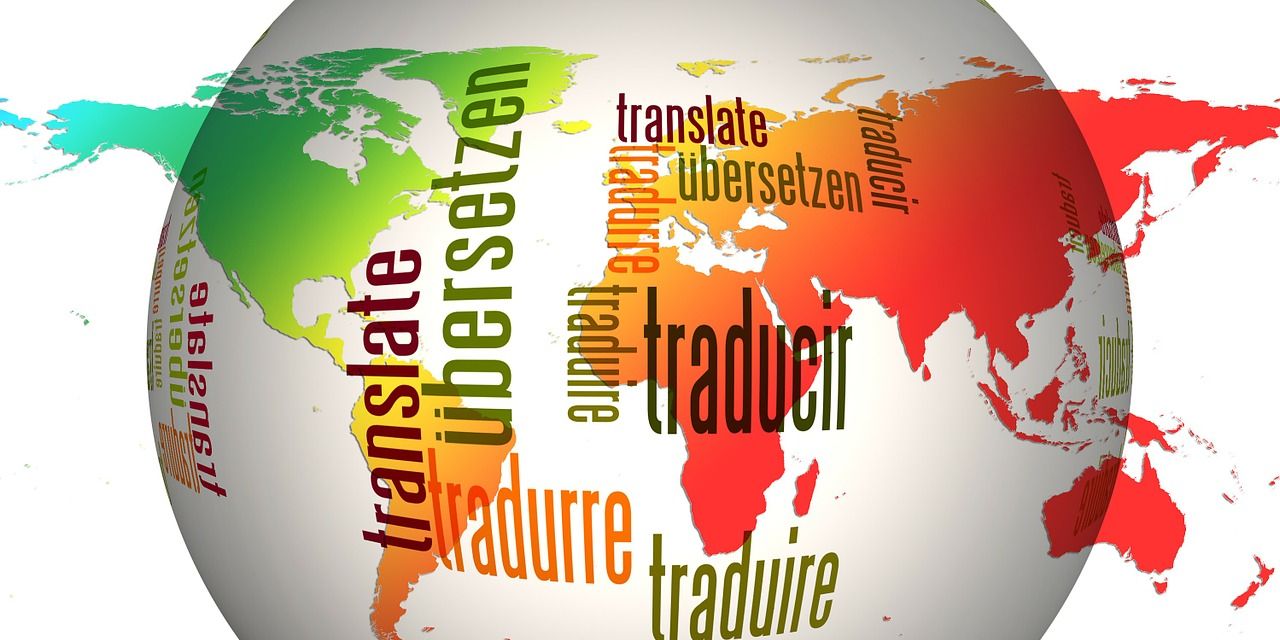
Cross System Translation
Algorithms to automatically map medical codes/terms between healthcare systems.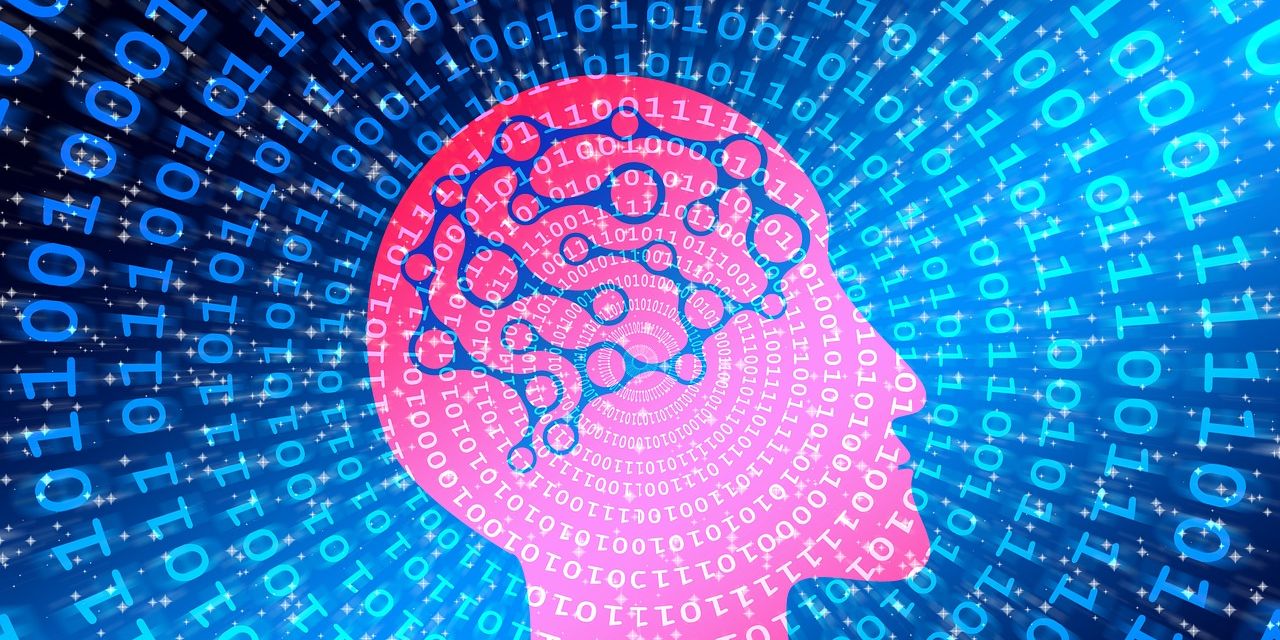
Automated Knowledge Extraction
Unsupervised methods for extracting medical knowledge from medical text and EHR data.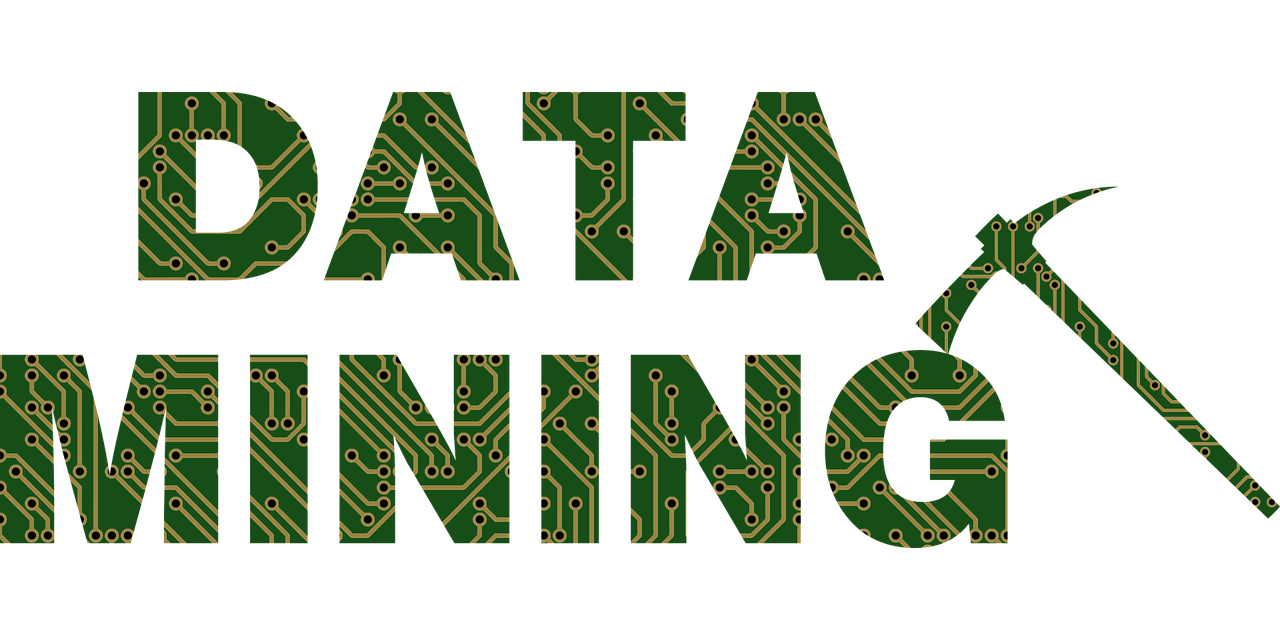
Text Mining
Nautural language processing software and tools to enable prediction modeling with textual data.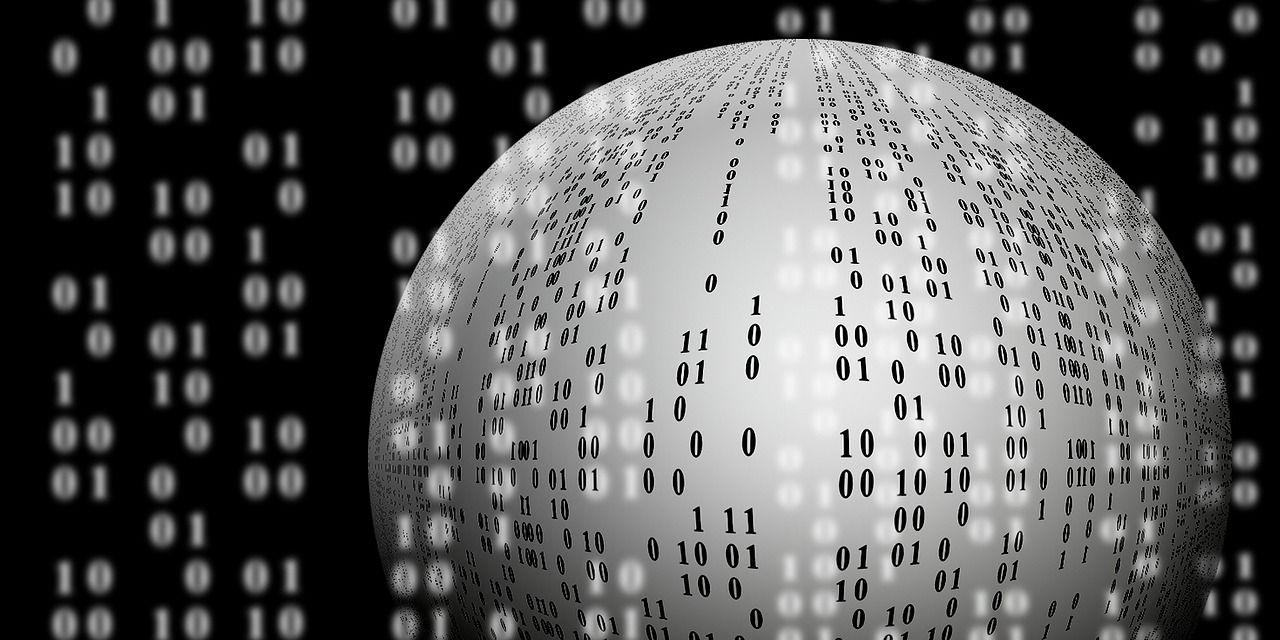
Transfer Learning
Statistical and machine learning methods for predictive modeling leveraging models built in other systems.Translational Clinical Research
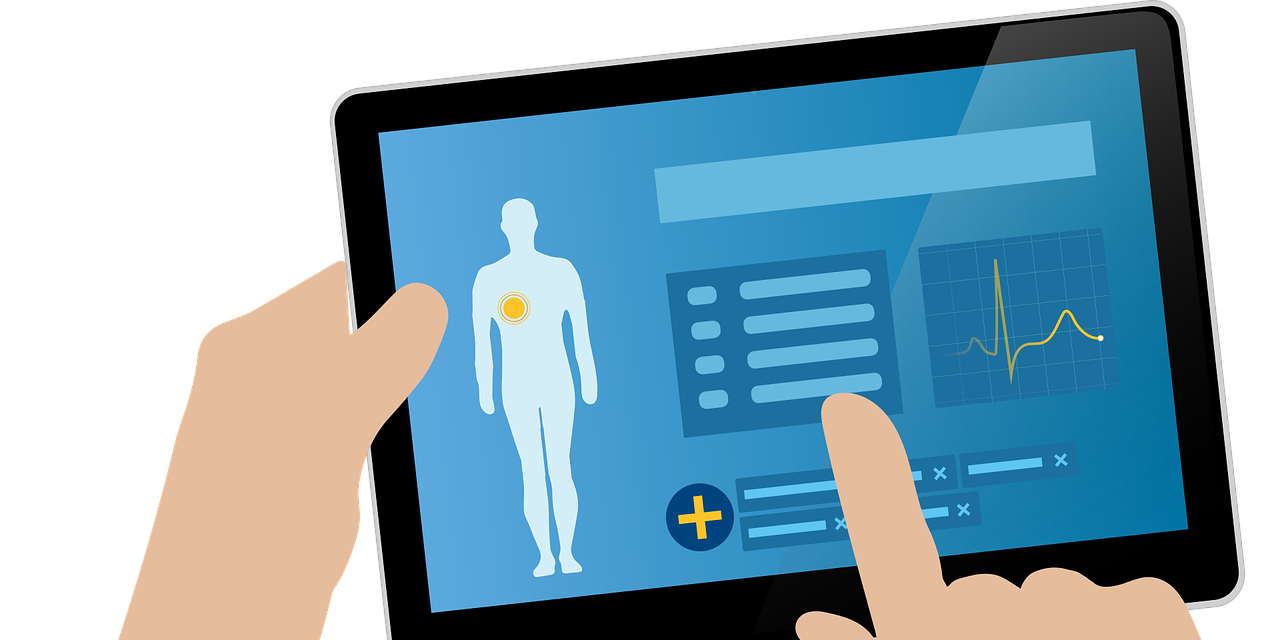
Enabling EHR for Research
Tools and algorithms to accurately automate annotation of clinical outcomes.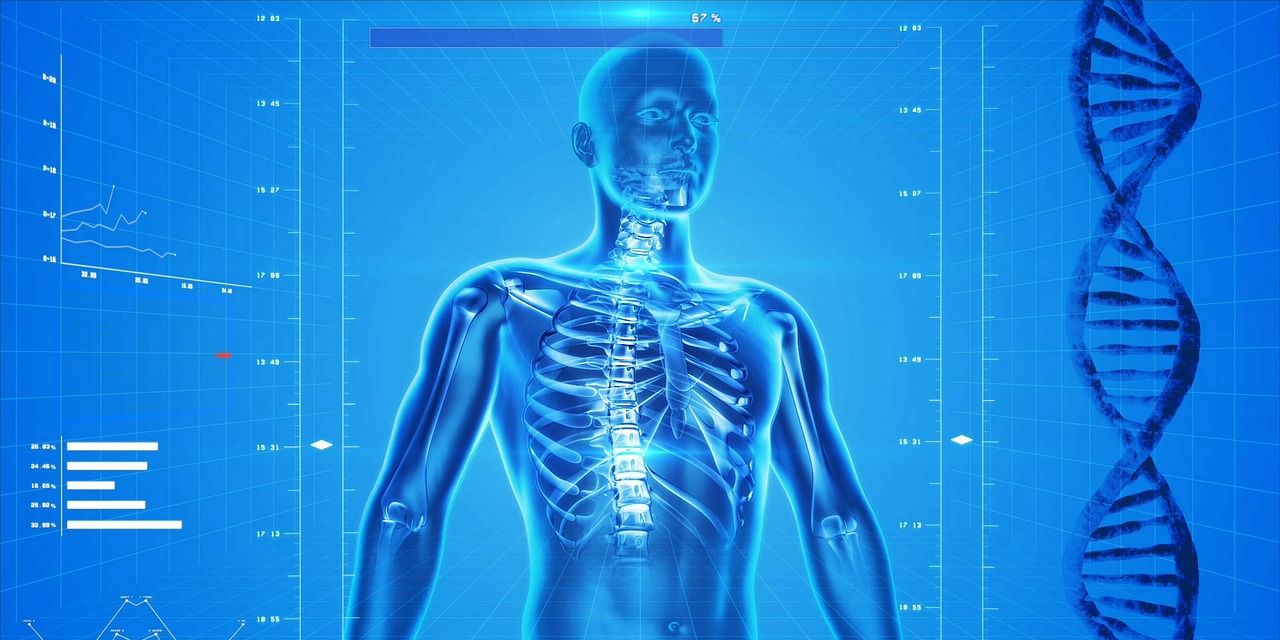
Biobank Linked EHR Analysis
Efficient and powerful genetic association analysis for discovery research.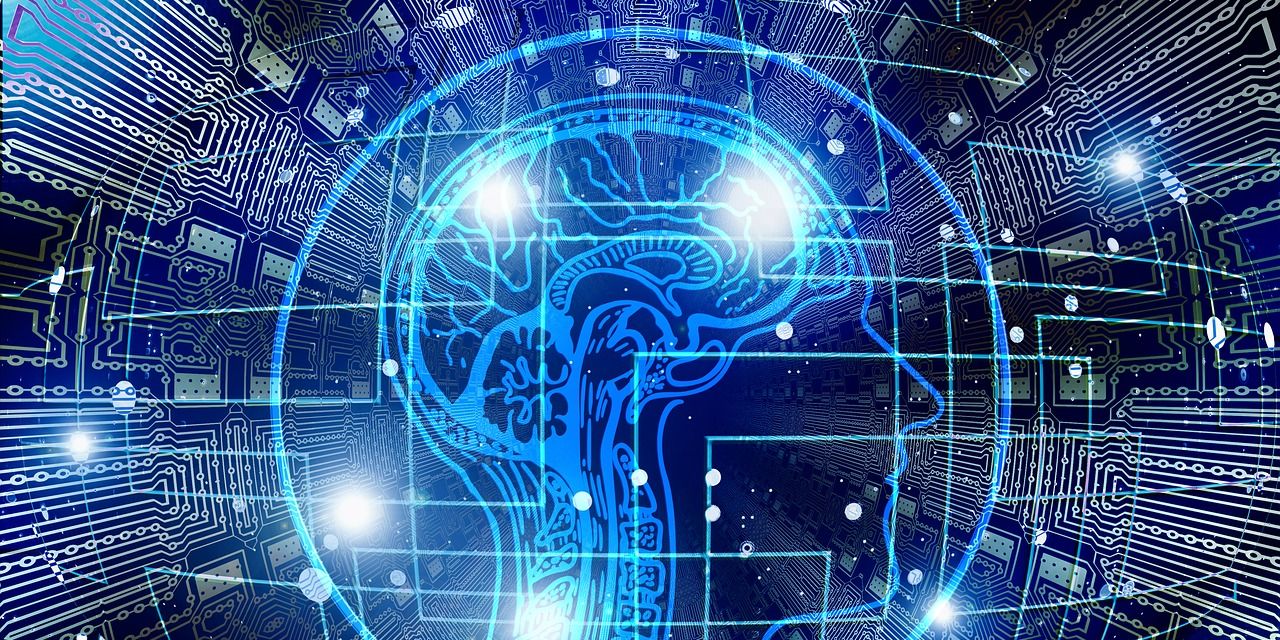
Learning Healthcare Systems
Extracting clinical knowledge and developing clinical decision support tools.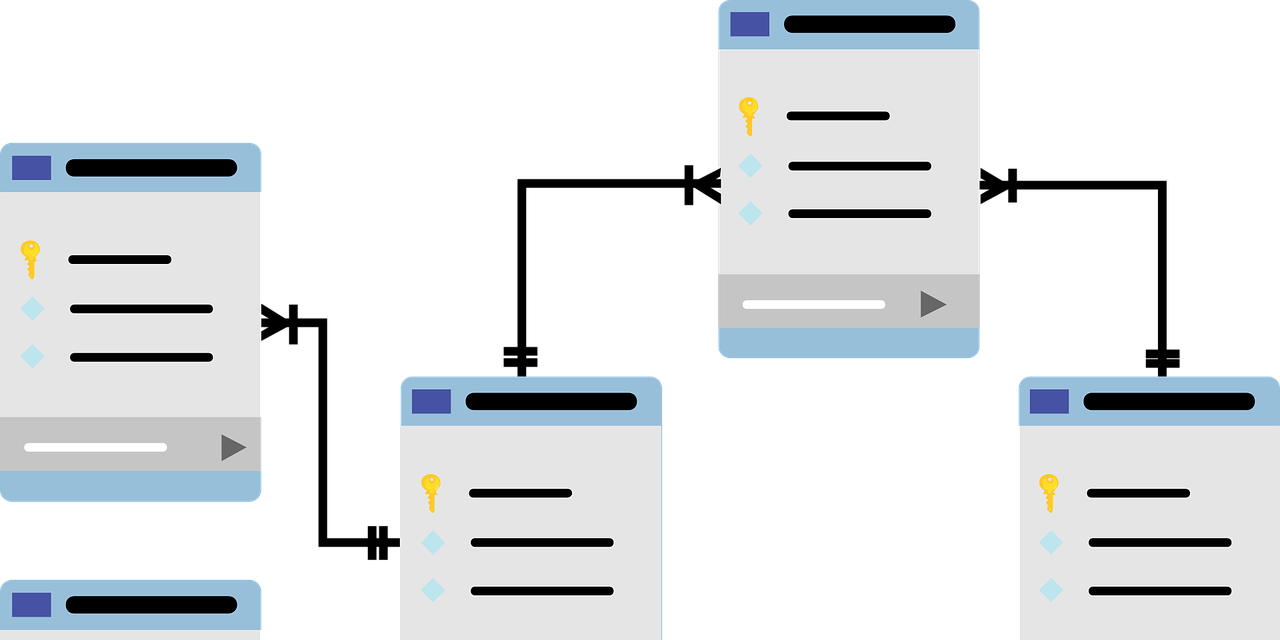
Implementation and Visualization
Algorithms and tools towards closing up the gap between research and practice.Big Data Analytics Core
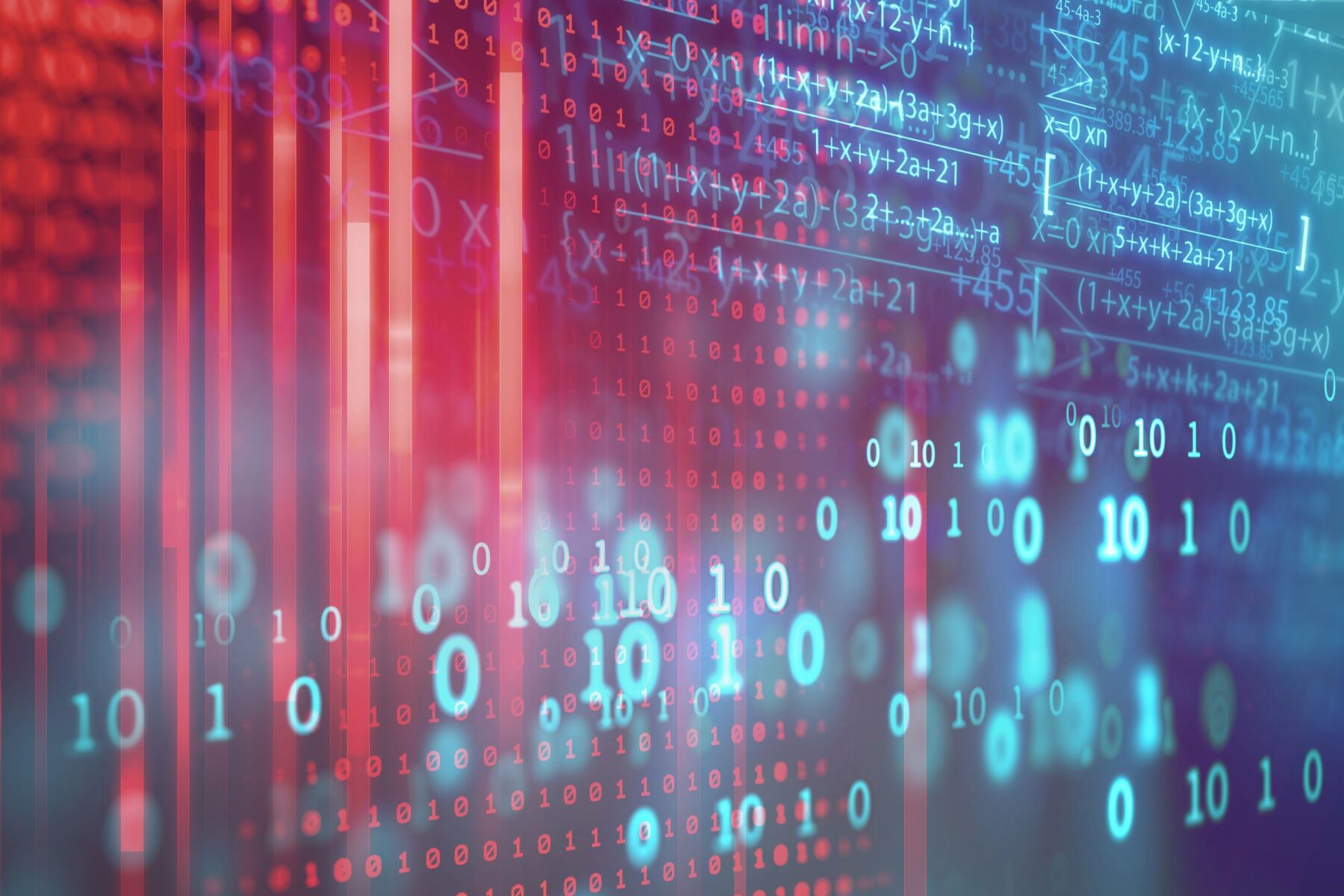